Analytical approach
Harnessing the power of cutting-edge machine learning models, our approach focuses on the correlation between crop yield and nutrient utilization, particularly Nitrogen (N), Phosphorus (P), and Potassium (K), as well as key biophysical factors that influence crop growth.
Once the machine learning model is trained, it can be deployed across scales to accommodate a wide array of NPK combinations and predict the expected yield against the desired NPK matrix.
These insights provide a foundation for optimizing fertilizer advice tailored to specific targets. This could include determining nutrient rates to achieve target yields or maximizing return on fertilizer investment, which can then be aligned with farmers’ investment capacity and risk tolerance.
This result can also aid in assessing nutrient requirements to mitigate agronomic yield gaps and estimate total fertilizer requirements nationally, thereby supporting decision-making in fertilizer purchase, distribution, and subsidy allocation.
Data Needs
This approach hinges on comprehensive field trial data encompassing a vast spectrum of NPK combinations and corresponding yield measurements, augmented by geospatial variables including soil properties, elevation, and climate data.
The crop yield data provides an understanding of yield effect due to nutrients added via fertilizers use, and the geospatial variables help further fine-tune these effects to locally relevant bio-physical factors.
Complexity
While of moderate complexity, this approach involves aggregating extensive field trial data, preparing geospatial layers, and constructing a predictive model.
The training and application of machine learning models such as gradient boosting, random forest, deep learning, and ensemble models are facilitated by semi-automated procedures in AgWise.
Exceptional data exploration skills, combined with agronomic knowledge and an understanding of machine learning models, are essential for assessing data quality, understanding its limitations, and making informed decisions in model selection.
The ground truth data is typically aggregated from numerous field experiments conducted across multiple years and institutes. It originates from a combination of on-farm and on-station trials, featuring various protocols such as mono- and inter-cropping, replicated and single treatments, as well as nutrient omission trials designed to test the effects of micronutrients, lime, and/or organic fertilizer addition.
Consequently, the data presents both strengths and challenges inherent in meta-analysis, necessitating meticulous data cleanup and exploration to mitigate error propagation that could undermine model accuracy.
While data quality challenges are common across approaches, this method uniquely grapples with model constraints tied to the nutrient levels and range present in the training dataset.
Specifically, the model’s capacity to estimate yield response is confined to the nutrient range covered by the training data. In instances where the NPK rates in the training dataset are not sufficiently broad for the target area, the recommendations generated from this approach may fall short of optimal levels, particularly in high-yield potential regions, potentially leading to missed opportunities for yield or profit maximization.
Additionally, transitioning from one nutrient level to the next, the resulting stepwise yield response curve poses a limitation. When the training data lacks diversity in NPK rates, this issue is exacerbated, resulting in a uniform yield response across a broader range of nutrient rates, contrary to biological norms
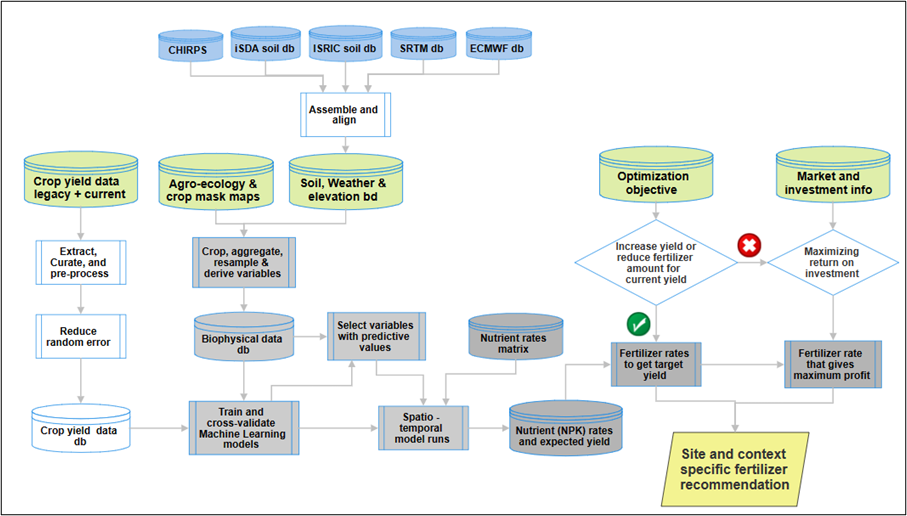